Sales data analysis examples provide real-world frameworks for extracting powerful insights from CRM data to boost revenue. Sales leaders rely on this in-depth analysis of their performance data to spot trends, identify issues, and inform strategy.
In this post, we will explore 10 impactful sales data analysis examples to showcase how you can unlock game-changing insights from your sales data. The goal is to provide sales leaders, analysts, and ops professionals with actionable sales data analysis strategies, frameworks, and techniques based on tangible examples.
Whether your objective is optimizing lead sources, predicting future trends, reducing churn, improving win rates, or maximizing marketing ROI – getting hands-on with your sales data certainly uncovers endless opportunities.
Why Sales Data Analysis Matters
Taking a deep dive into your sales performance data uncovers all kinds of handy revelations. From top-performing products to marketing channels that are proving to be more valuable, there’s a plethora of opportunities. Here are some of the benefits of sales data analysis:
- Finding Your Most Valuable Customers – You can identify key traits of your best customers like higher order frequency or bigger deal sizes. Use this intel to find more prospects that look just like them!
- Seeing What Marketing Channels Really Work – Analyze your marketing performance data to see which channels drive the most and highest quality leads. Then get more bang for your buck by focusing your budget on what works.
- Pinpointing Sales Process Potholes – Track results at each sales funnel stage to spot where deals are stalling out. Address problem areas to smooth out the path from lead to customer.
- Improving Forecasting – Review historical sales patterns and external factors to build statistical models that more accurately predict future performance.
- Tracking Rep Performance – Maintain transparency by looking at how each salesperson’s key metrics stack up against goals. Identify all-stars and struggles.
Sales Data Analysis Process Overview
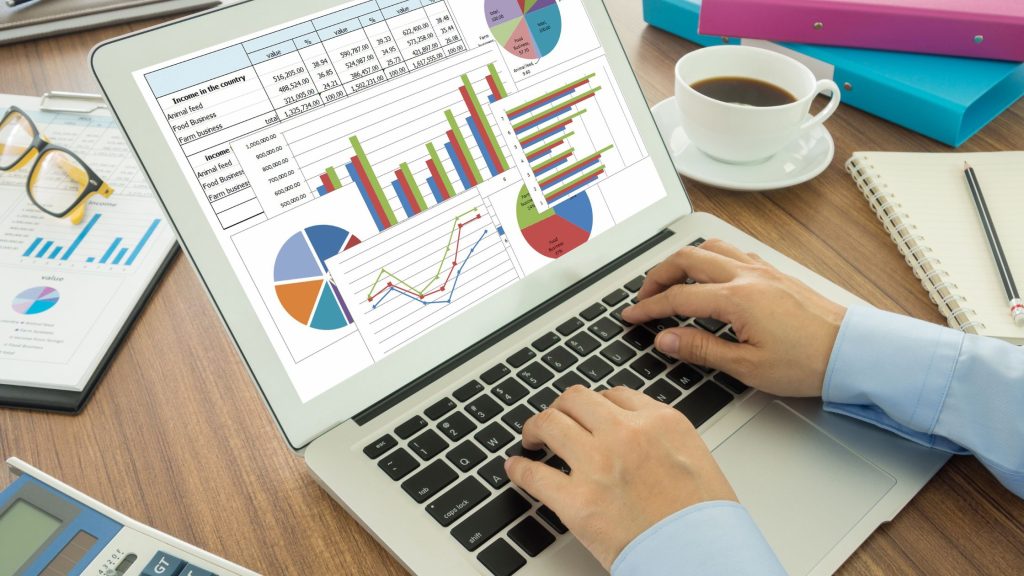
Sales data analysis isn’t something that just magically happens. Every effective sales analysis follows a defined process. That process might differ based on the tools and data collection mechanisms in your workflow, but the general process mostly includes the following:
- Identify your goals – Develop specific questions you want the data to answer.
- Collect relevant data – Compile applicable sales data from your CRM, marketing platforms, and other sources.
- Clean and organize – Structure your data in an analysis-friendly format.
- Conduct analysis – Dive deep using calculations, visualizations, statistical modeling, and other techniques.
- Interpret insights – Determine meaning from the analysis results.
- Make data-driven decisions – Take strategic action based on analytical insights.
Now let’s look at impactful sales data analysis examples in action across these key areas:
Sales Data Analysis Examples
1. Customer Segmentation Analysis
Customer segmentation analysis involves dividing customers into tiers such as high-value, mid-value, and low-value based on their purchase history, order frequency, recency, and lifetime spending amounts. The goal is to define the key characteristics of your best and most profitable customers, such as larger average order sizes or higher purchase frequency. These insights allow you to develop targeted marketing campaigns and outreach strategies tailored specifically to each customer segment’s needs and behaviors. For example, you may provide special promotions, discounts, and premium perks to high-value segments in order to focus energy on the customers that generate the most revenue.
2. Lead Source Analysis
One of the most common sales data analysis examples’ is lead source analysis. Lead source analysis focuses on tracking important metrics such as conversion rate, average deal size, sales cycle length, and marketing ROI for each lead source across campaigns.
The goal is to identify your best-performing lead sources that convert at the highest rates and result in the fastest sales cycles and sales team productivity. You can then optimize spending by reducing the budget on poorer-performing lead sources with lower conversion rates and shift that spending to sources generating a higher output of qualified leads more efficiently. This level of lead source optimization improves overall lead quality.
3. Sales Funnel Analysis
Sales funnel analysis involves mapping out each stage of your sales funnel from the initial lead all the way to the closed deal. You then attach historical performance data to each step of the funnel, analyzing conversion percentages, cycle times, and bottlenecks. Comparing performance by the sales team allows you to see who excels best at different stages of the funnel. Recommendations can then be made to optimize underperforming stages through changes to workflow, staffing, or implementing automation tools. The overall goal is to increase conversion rates and speed at each sales funnel stage, which directly translates into higher revenue.
4. Churn Analysis
The goal of churn analysis is to identify the customers that are most likely to churn by looking for declining purchase frequency, increasing support tickets, expiring subscriptions, or other signals. Developing leading indicators that precede churn, such as late or missed payments and feature downgrades, allows you to get ahead of it. Proactive retention campaigns with special offers and incentives can then be targeted at these at-risk accounts detected early. Reducing customer churn retains revenue while cutting the costs of acquiring new customers.
5. Win/Loss Analysis
Win/loss analysis involves comparing won and lost deals across factors like lead source, product, industry, sales rep, deal size, and other attributes. The goal of this type of sales data analysis is to identify patterns and commonalities among won deals that can be replicated as best practices. Taking note of frequent commonalities in lost deals allows sales strategy, product offerings, pricing, and messaging to be adjusted accordingly. These win/loss insights derived from historical deal data optimize sales tactics to be more effective at closing deals.
6. Sales Forecasting Analysis
The goal of sales forecasting analysis is to build models capable of predicting future revenue and the number of deals closed. The models incorporate historical performance data, seasonality patterns, market conditions, and other variables. By continually analyzing discrepancies between sales projections and actual results, the forecast models can be refined over time to improve accuracy. More accurate revenue and pipeline forecasts allow leadership to set realistic targets and inform strategic budget planning.
7. Competitive Analysis
The competitive analysis focuses on thoroughly researching competitor product features, pricing, promotions, and messaging strategies in your market. By comparing competitor offerings to your own, you can identify competitive advantages, weaknesses, and gaps. These insights allow you to adapt your sales and marketing strategies accordingly. For example, your product roadmap, pricing structure, and sales collateral can be adjusted to differentiate from competitors. Keeping a pulse on the competitive landscape is key to aligning go-to-market strategies.
8. Price Elasticity Analysis
Price elasticity analysis involves testing different pricing for sample customer segments and product tiers. By analyzing the impact of price changes on deal size, win rates, and overall revenue, you can determine price sensitivity and willingness to pay. These insights allow you to optimize pricing strategy for profitability based on empirical data rather than guesswork. The end goal is to maximize revenue potential through data-driven pricing.
9. Sales Rep Performance Analysis
Sales rep performance analysis tracks individual team members against key performance metrics and goals. Comparing cohorts’ sales activities, close rates, deal sizes, and other KPIs identifies high performers for rewards and development areas for struggling reps. Providing customized coaching and incentives drives motivation and enables management to align skill sets for success. This analysis provides key visibility into sales team effectiveness.
10. Campaign Analysis
Campaign analysis focuses on measuring the tangible sales pipeline and revenue generated from marketing campaigns and assets. By determining marketing ROI, you can double down on the campaigns, channels, and activities demonstrating the best measurable results. Low-performing campaigns can be eliminated and the budget reallocated to what works. This analysis ensures marketing spend is optimized to drive sales impact.
How to Gain Value from Sales Data Analysis Examples
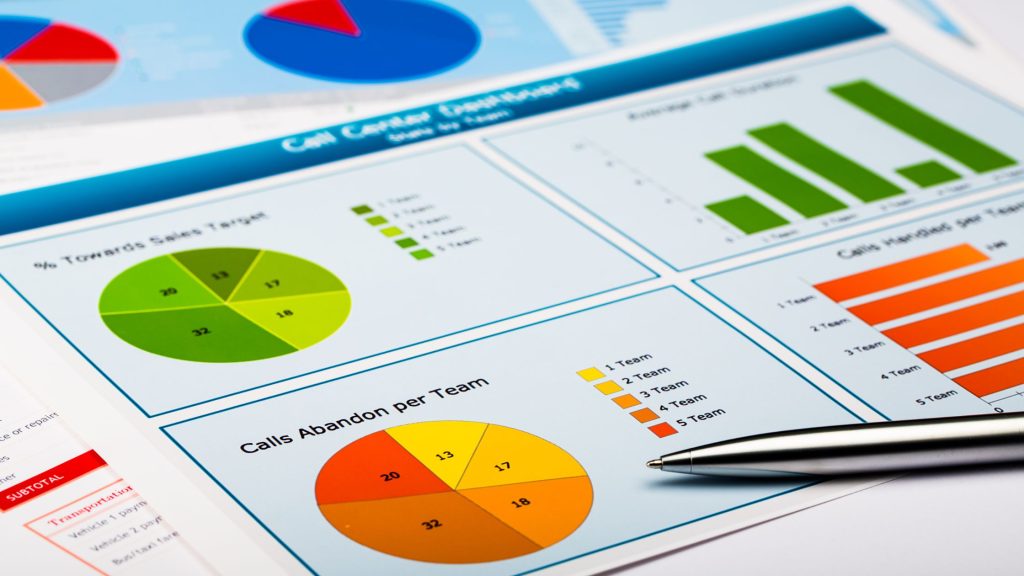
The key to gaining value from sales data analysis is translating insights into strategic action. Here are some ways to apply analytical findings:
- Target outreach to high-value segments
- Shift marketing budget to productive lead sources
- Fix broken parts of the sales funnel
- Retain customers with high churn risk
- Train sales team on winning sales tactics
- Incentivize sales behaviors that drive revenue
- Right-size forecasts to inform budget planning
- Set data-backed pricing for each customer profile
The possibilities are endless when you let data guide your sales strategy.
Start Uncovering Your Sales Insights
Follow the examples and best practices outlined here to start uncovering data treasures that spark sales growth. Also, consider incorporating some AI tools for sales into your workflow to make this analysis process more automated. And let data-driven decisions be your competitive advantage. The insights are waiting to be discovered in your existing sales data. Unleash its potential today!